Contents
Building advanced AI systems isn’t just about feeding them with a vast amount of training data—quality matters just as much as quantity. Models trained on inaccurate or biased data don’t just underperform; they lose stakeholders’ trust, raise ethical concerns, and hinder business outcomes. In fact, a recent study reveals that poor data quality is one of the key reasons behind the failure of AI initiatives, often costing companies up to 6% of their annual revenue. To avoid these setbacks, investing in a data cleansing process is crucial.
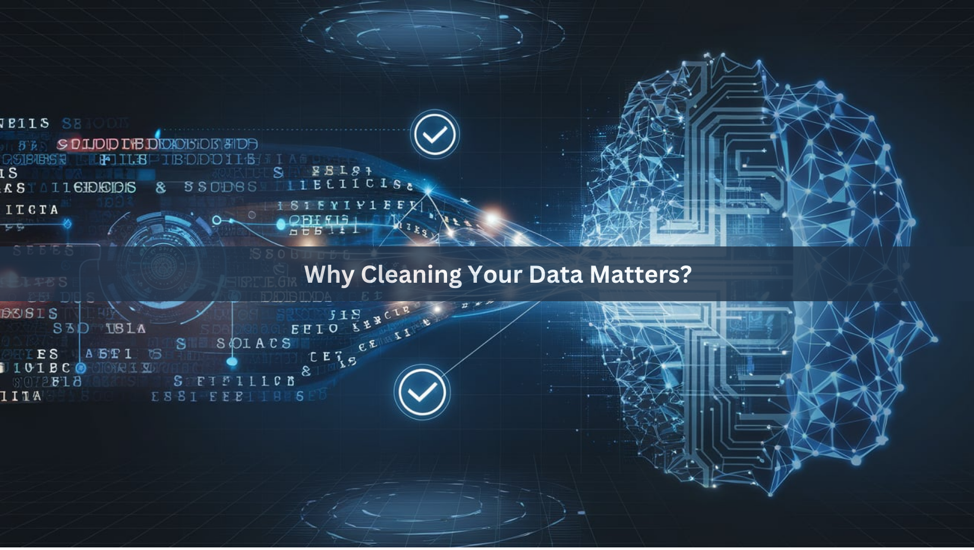
i
Let’s understand through this blog how prioritizing data cleaning can help organizations develop more reliable and efficient AI models that can handle complex real-world scenarios with precision.
Why is Dirty Data a Big Problem for AI – Its Impact & Consequences
Before jumping directly to data cleansing practices, let’s first understand how neglecting data quality during initial AI model development and training can cause big trouble. Here’s what can go wrong when AI models are trained on low-quality data:
● AI Models Can’t Think beyond Data Bias
When AI training data is incomplete or biased (not representing real-world scenarios), it leads to discriminatory outcomes, favoring one population over others. This not only limits the model’s ability to generalize but also raises serious concerns about its fairness, ethics, and reliability.
Real-world example:
In their recent analysis, Bloomberg found that renowned OpenAI’s GPT tool also reflects racial bias in its outcome when used for recruitment purposes. The company conducted an experiment and asked GPT to rank the resumes 1,000 times. To their surprise, GPT 3.5 showed a clear preference for certain demographics over others.
Resumes with names typically associated with Black Americans were consistently ranked lower for financial analyst roles, while those with names linked to other racial or ethnic groups had better odds of making the top cut. The bias was significant enough to fall short of standards used to detect discrimination against protected groups, raising serious concerns about fairness in AI-powered hiring tools.
● Garbage In, Garbage Out – No Intelligence without Accuracy
The intelligence and accuracy of AI systems come from their training data. If the data feeding into an AI model is dirty (consists of duplicates, inconsistencies, or outdated information), the system won’t be able to generate meaningful insights or accurate outcomes. This will not only reduce the model’s reliability but also increase the need for constant re-training.
● Wasted Resources on Endless Debugging and Fixes
Dirty data leads to false positives, misclassifications, and errors that require manual interventions by subject matter experts. To identify the root cause of data errors and rectify them to improve the quality of training datasets, companies have to allocate a dedicated team of professionals, which eventually impacts their operational efficiency.
● Training Bottlenecks Leading to Project Delays
A critical factor behind delays in AI projects is the presence of unstructured or mislabeled datasets. When datasets are messy or incorrectly labeled, it slows down the training process because the model struggles to identify meaningful patterns. More time and iterations are required by data labeling experts to achieve acceptable performance, pushing the AI model’s development and deployment timeline further.
How Data Cleaning and Validation Ensures AI Readiness
To eliminate subtle biases from the labeled datasets and ensure that the AI model doesn’t inherit hidden flaws from the data, it is crucial to clean and validate the information before it undergoes the annotation process. This is how data cleansing and validation can improve AI efficiency and accuracy:
Identifying Duplicates, Anomalies, and Outliers: When duplicate entries or outliers are available in the training dataset, overfitting occurs as the AI model starts giving undue importance to repeated or unnecessary details.Data cleaning helps identify outliers, duplicates, and anomalies that could distort model behavior, ensuring the AI remains adaptive and accurate over time.
Standardizing Data across Sources: When data flows in from multiple sources—like APIs, databases, or IoT sensors—validation ensures everything aligns under a common structure, preventing data errors during model training.
Mitigating Biases Before They Creep in: Early detection of imbalances in training data through data validation minimizes the risk of bias perpetuation in the model’s learning phase.
Enhancing Predictive Accuracy: A clean, validated dataset means fewer noisy inputs. This enables the AI model to focus on meaningful correlations and deliver more accurate predictions.
Ensuring Compliance and Governance: Continuous data checks also verify that AI model training data meets regulatory standards, ensuring compliance with GDPR, HIPAA, or other industry-specific guidelines.
Role of Automation in Data Cleaning for AI
While data cleaning is an absolute necessity to ensure the AI model’s reliability, scrubbing and validating a vast amount of training datasets is time-intensive. Through automation, you can reduce time-to-market and ensure efficiency. Automated data cleaning not only helps you save time but also:
● Facilitates Real-time Data Validation
Automated data cleansing tools such as OpenRefine, Winpure, and Talend validate voluminous training data against pre-set rules, flagging errors in real time to avoid disruptions in the AI pipeline.
● Ensures Seamless Scalability
The most significant benefit of these tools is that they can handle both – millions of data points and diverse data sources, adapting seamlessly to your scalability needs while maintaining consistency and efficiency.
● Improves Resource Allocation and Cost Efficiency
As automated data cleansing tools process large amounts of data within seconds, you can strategically allocate your resources for other operations, enhancing overall efficiency and reducing labor costs.
Do Not Overlook the Human Element in Data Cleaning
Data cleaning for AI is a context-driven, iterative process for which you cannot rely solely on automation. While automated data cleaning tools can significantly reduce processing time, they cannot replace the nuanced judgment that only humans provide. Algorithms can often miss some outliers or inconsistencies during the data cleansing process, which human experts can identify and fix.
Subject matter experts ensure that data isn’t just clean but meaningful and aligned with real-world scenarios. Hence, by integrating the expertise of data professionals with the efficiency of automated tools, you can facilitate improved error handling, prevent overlooked biases, and maintain the integrity required for AI models to perform reliably.
Final Verdict – The Path to Accurate AI Begins with Clean Data
In the current tech-driven era, where AI’s reliability and success depend on the quality of its training datasets, effective data cleaning becomes non-negotiable. Implementing a rigorous data cleansing process not only sharpens model accuracy but also minimizes costly errors.
However, as datasets grow in size and complexity, managing the process in-house can strain resources and introduce operational inefficiencies. To stay scalable and efficient, businesses can consider outsourcing data cleansing services. By bringing in specialized expertise to handle large volumes of data with precision, organizations can perform at their best without diverting focus from core business operations.